H|O|T Speech Metric
What is the H|O|T Speech Metric?
What can you do with it?
Compare the “temperature” of Reddit, X, and YouTube
Check the reactions to a news event
Check the reactions to a change at Reddit, X, or YouTube
See content from each platform with especially H|O|T (or not H|O|T) comments
See sample comments for particular stories
Computing the H|O|T Speech Metric
Deep Dive
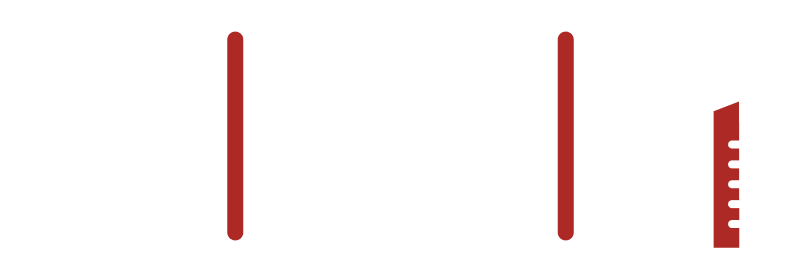
H|O|T Speech Metric
(Hateful, Offensive, Toxic)
Loading...
What is the H|O|T Speech Metric?
We collect social media comments about top news stories.
The H|O|T Speech Metric is a calibrated estimate of the percentage
of those comments that people would label as Hateful, Offensive, or Toxic.
Higher scores are worse!
What can you do with it?
Here are a few examples of ways you can use the H|O|T Speech Metric.
You can do your own analyses and create graphics like these on our
deep dive page.
Compare the “temperature” of Reddit, X, and YouTube
Loading...
Consistently, YouTube had the H|O|Ttest comments and reddit the least, with X (Twitter) falling between.
Check the reactions to a news event
Loading...
On February 24, 2022, Russia invaded Ukraine. While there was an immediate uptick in H|O|T comments,
particularly on YouTube, in the week or two after the invasion,
the general temperature of social media comments on top news stories did not seem to be noticeably higher
thereafter when compared to pre-February 24 scores.
Check the reactions to a change at Reddit, X, or YouTube
Loading...
Elon Musk announced his Twitter acquisition bid on April 14, 2022;
media reports of the deal began on April 23; and the deal officially closed on October 27.
Since Musk’s acquisition, there have been sporadic reports of increased hate speech, for example,
on X, but we haven’t seen a significant uptick in the H|O|T Speech scores related to
top news stories during that time (October 2022 - June 2023). However, for that same time period, the
Iffy Quotient
went up significantly on X even though the H|O|T Speech scores didn’t.
See content from each platform with especially H|O|T (or not H|O|T) comments
Loading...
These are the stories with the highest H|O|T Speech scores on Reddit for the week ending on October 29, 2023.
The two H|O|Ttest stories are about Donald Trump and the Biden family.
Other story topics with H|O|T comments include other politicians (both U.S. and non-U.S.),
bomb threats to schools, a mass shooting in Maine, and sexual abuse in the Catholic church.
See sample comments for particular stories
Source: ...
...
Loading data ...

In this story about a 12-year-old child who emailed bomb threats to local schools,
the H|O|T comments typically feature expletives used in various ways,
sometimes generically and other times more directly and aggressively.
Some of the H|O|T comments also denigrate a particular target—for example,
the child at the center of the story or local legislators.
Computing the H|O|T Speech Metric
To calculate the H|O|T Speech Metric for a platform and time period, we:
- Collect a set of comments from mainstream social platforms;
- Query NewsWhip for the 1,000 most engaged-with URLs each day, on Facebook and X (formerly Twitter) each, where the URLs are sorted based on an engagement metric tracked by NewsWhip (e.g., likes, comments, and replies for Facebook; tweets and retweets for X).
- Classify each URL as “hard news” or “soft news,” based on the URL’s headline and summary, and keep only the hard news URLs.
- Collect social media posts talking about those hard news URLs on Reddit, X, and YouTube, as well as comments under those posts. For each URL, sample an equal number of comments from all three platforms.
- Obtain classifier scores (from Jigsaw’s Perspective API) for those comments;
- Pass those classifier scores to our prevalence estimator function, which predicts the percentage of comments that people would label as H-O-T. This prediction is the H|O|T Speech Metric for that time period.
- Sample comments for a base time period from the platform
- Have people label those comments (the training sample) as H-O-T
- Get classifier (Perspective API) scores for those comments
- Compare human labels with classifier scores to generate the calibration curve function
Deep Dive
We have a page with a suite of related analytical tools
that unlocks the H|O|T Speech Metric’s power to illuminate comparisons over time and between platforms.
You can use that page to create customizable graphics like all of those shown on this page,
for any time period since the week ending July 18, 2021.